.jpg)
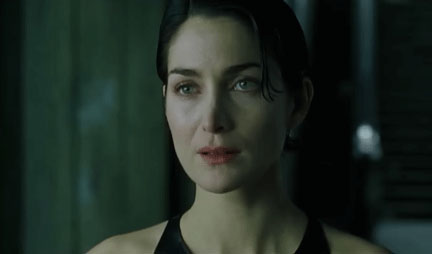
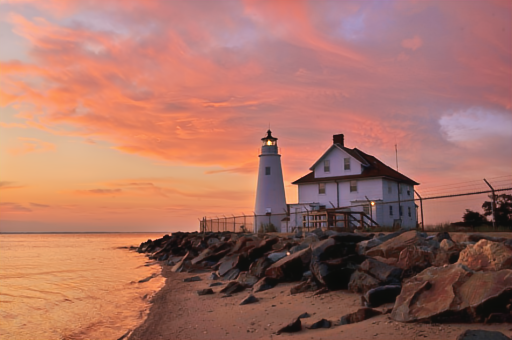
.png)
Existing low-light image enhancement (LIE) methods have achieved noteworthy success in solving synthetic distortions, yet they often fall short in practical applications. The limitations arise from two inherent challenges in real-world LIE: 1) the collection of distorted/clean image pairs is often impractical and sometimes even unavailable, and 2) accurately modeling complex degradations presents a non-trivial problem.
To address the aforementioned challenges, we introduce a novel training-free and unsupervised framework, named AGLLDiff, for real-world low-light enhancement. In contrast to prior works that predefine the degradation process, our approach models the desired attributes and incorporates this guidance within the diffusion generative process. As shown in Fig. 1, noisy images are degradation-irrelevant conditions for the DM generative process. Adding extra gaussian noise makes the degradation less distinguishable compared with its corresponding reference distribution. Since diffusion prior can serve as a natural image regularization, one could simply guide the sampling process with easily accessible attributes such as image exposure, structure and color of normal-light images.
By constraining a reliable HQ solution space, the core of our philosophy is to bypass the difficulty of discerning the prior relationship between low-light and normal-light images, thus improving generalizability.
The overview of AGLLDiff is illustrated above. Given a degraded low-light image y0 in the wild domain, the diffusion forward process adds a few steps of slight Gaussian noise to the y0, aiming to narrow the distribution between the degraded image and its potential counterpart, i.e., the HQ image. After obtaining the noisy image xt, we implement the reverse generative process through a pre-trained diffusion denoiser and attribute guidance to generate the enhancement result x0. The inherent attributes of normal-light samples, such as image exposure, structure and color, can be readily derived from their degraded counterparts. Further elaboration on the pivotal components of our method, including attributes, classifiers, and targets, is provided in subsequent sections.
@misc{lin2024aglldiff,
Author = {Yunlong Lin and Tian Ye and Sixiang Chen and Zhenqi Fu and Yingying Wang and Wenhao Chai and Zhaohu Xing and Lei Zhu and Xinghao Ding},
Title = {AGLLDiff: Guiding Diffusion Models Towards Unsupervised Training-free Real-world Low-light Image Enhancement},
year ={2024},
eprint ={2407.14900},
archivePrefix={arXiv},
primaryClass={cs.CV},
}